Machine Learning Approaches for Automated Mental Disorder Classification based on Social Media Textual Data
Keywords:
Machine learning, Mental disorders, Text data, Reddit, NLP, BPD, BipolarAbstract
The application of machine learning models to mental health-related text data offers a novel approach to discern patterns and trends, aiding in the identification of subgroups and personalized treatment options. This research explores the classification of mental disorders based on text data extracted from subreddits focused on mental health. The dataset consists of 10,000 rows of text collected from four subreddits: 'BPD', 'bipolar', 'depression', and 'Anxiety', along with a combined category 'others' encompassing 'mentalillness' and 'schizophrenia'. To enable the application of machine learning models, various text preprocessing techniques were applied, including the removal of URLs, punctuation marks, and stopwords, as well as the transformation of raw text documents into a matrix of TF-IDF features. These preprocessing steps were performed on both the titles and text contents of the posts. Three machine learning models, namely Multinomial Naive Bayes, Multi-layer Perceptron, and LightGBM, were employed for the classification task. The models were trained and evaluated separately on both the post titles and the text content. The accuracy of each model was assessed to measure their performance. The results indicate that the Multinomial Naive Bayes model achieved an accuracy of 0.706 when classifying based on titles, while the accuracy increased to 0.73 when classifying based on the text content. The Multi-layer Perceptron model yielded an accuracy of 0.68 for title classification and 0.714 for text content classification. Notably, the LightGBM model exhibited superior performance, achieving an accuracy of 0.724 when using titles for classification, and an even higher accuracy of 0.77 when employing the text content. This research demonstrates the efficacy of machine learning models in classifying mental disorders using text data extracted from social media. These findings contribute to the ongoing exploration of using social media data for mental health analysis and may aid in developing automated tools for early detection and support for individuals facing mental health challenges.
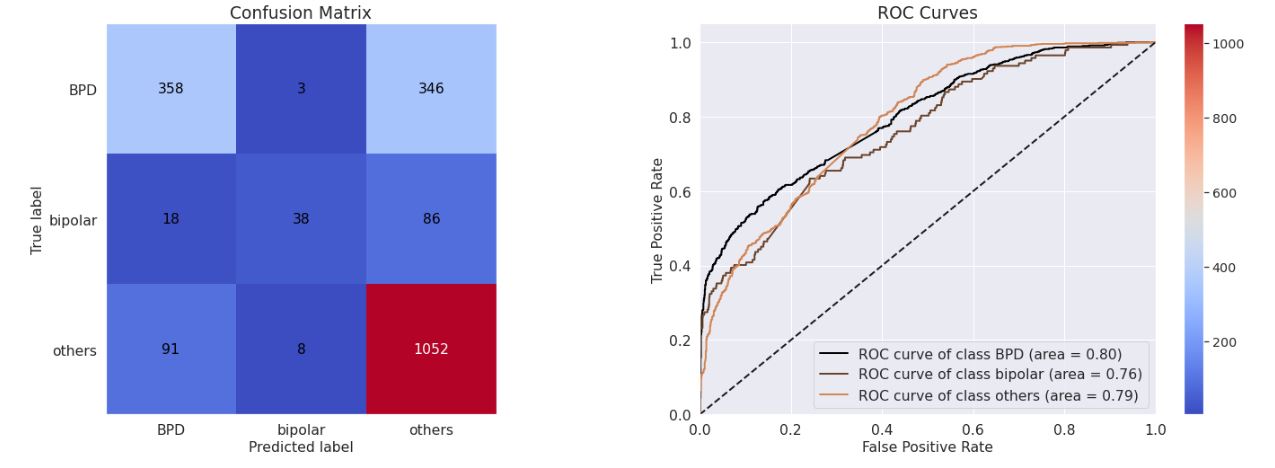