Patient-Centric Ethical Frameworks for Privacy, Transparency, and Bias Awareness in Deep Learning-Based Medical Systems
Keywords:
Medical Ethics, Deep Learning, Privacy, Transparency, Bias, Deep Learning EthicsAbstract
The rapid advancement and deployment of deep learning-enabled medical systems have necessitated the development of robust ethical frameworks to address potential challenges and pitfalls. Based on the foundational principles of medical ethics—non-maleficence, beneficence, respect for patient autonomy, and justice—three ethical frameworks are proposed in this study for the deployment and oversight of deep learning systems in healthcare. This study presents these three distinct yet interconnected ethical frameworks focusing on patient privacy, transparency, and bias mitigation. The patient privacy framework argues for the importance of patient autonomy. It advocates for informed consent, emphasizing the need for patients to be apprised of the system's workings, benefits, potential risks, and alternatives. Consent should be voluntary, devoid of implicit coercion, and patients must retain the right to revoke it without repercussions. The framework also included the principles of transparency, beneficence, privacy, continual consent, accessibility, and accountability. It champions the idea that consent is dynamic, necessitating regular updates, especially when significant system changes occur. Our ethical framework for transparency accentuates the need for full disclosure. Stakeholders should be provided with a general overview of the system's operations, its inputs, and decision-making processes. Performance metrics, including accuracy, sensitivity, and specificity, should be transparently communicated. Openness, through open-source initiatives and third-party audits, is promoted. The principles of accountability, data transparency, continuous improvement, inclusivity, and external validation are also made integral to this framework, ensuring that stakeholders are consistently informed and engaged. The bias minimization framework highlights the imperative of awareness. Stakeholders should be educated about potential biases and their ramifications. The system should be regularly evaluated for inherent biases, both overt and subtle. Representation is crucial; training data must reflect diverse populations, considering various demographic factors. This framework also promotes fairness, ensuring equitable system performance across different patient groups. Transparency in bias reporting, accountability in bias correction, continuous monitoring, inclusivity in stakeholder engagement, and collaboration with interdisciplinary teams are also included and discussed.
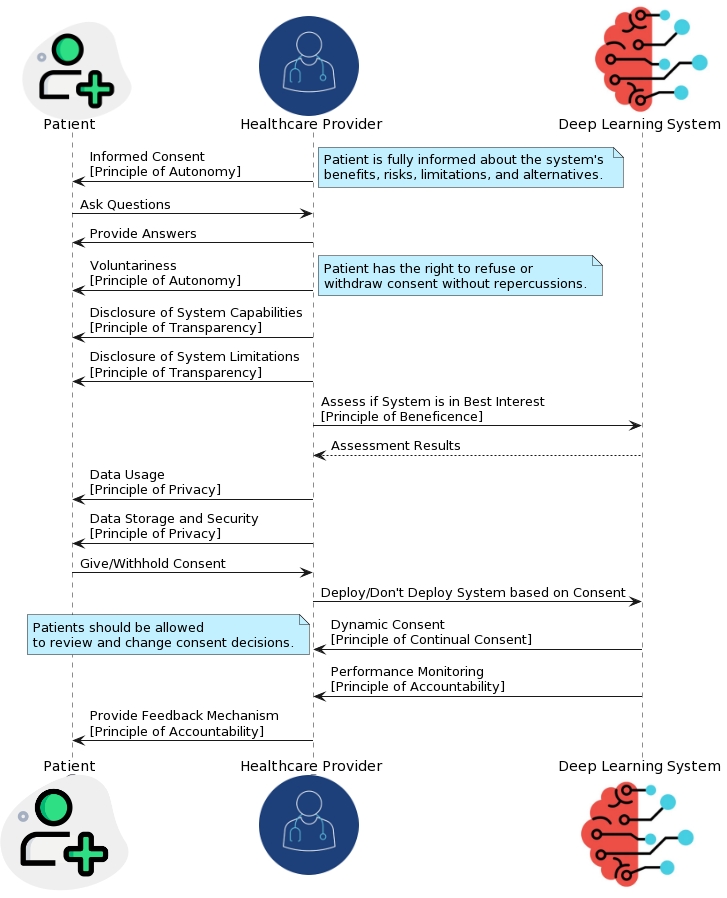
Downloads
Published
How to Cite
Issue
Section
License
Copyright (c) 2020 Applied Research in Artificial Intelligence and Cloud Computing

This work is licensed under a Creative Commons Attribution-NonCommercial-NoDerivatives 4.0 International License.