Employing Deep Learning for Automated Inspection and Damage Assessment in Civil Infrastructure Systems
Abstract
The integrity of civil infrastructure systems, including bridges, roads, tunnels, and buildings, is critical for public safety and economic stability. Traditional methods of inspection and damage assessment often rely on manual visual inspections, which can be time-consuming, subjective, and prone to errors. With advancements in deep learning, there is an opportunity to revolutionize the inspection and damage assessment processes through automated systems that offer increased accuracy, efficiency, and scalability. This paper explores the application of deep learning for automated inspection and damage assessment in civil infrastructure systems. We analyze various deep learning techniques, including Convolutional Neural Networks (CNNs), Recurrent Neural Networks (RNNs), and Generative Adversarial Networks (GANs), and their roles in defect detection, damage classification, and structural health monitoring. We also discuss the challenges associated with implementing these technologies, such as data quality, model interpretability, and integration with existing infrastructure. By addressing these challenges, deep learning can significantly enhance the capabilities of automated inspection systems, leading to more reliable and timely assessments of infrastructure health.
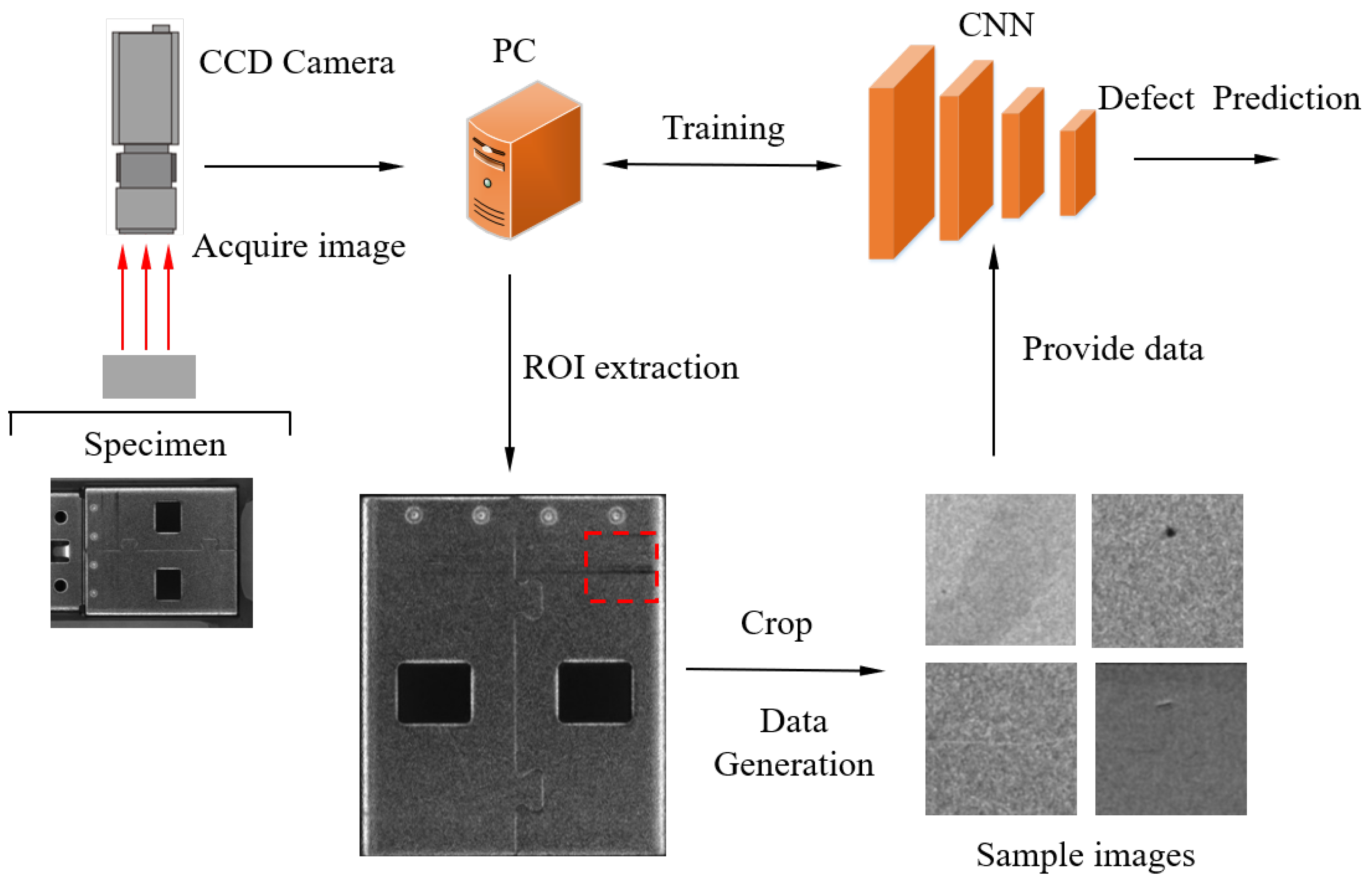
Downloads
Published
How to Cite
Issue
Section
License
Copyright (c) 2024 Applied Research in Artificial Intelligence and Cloud Computing

This work is licensed under a Creative Commons Attribution-NonCommercial-NoDerivatives 4.0 International License.