Enhancing Autonomous Vehicle Performance through Edge Computing: Technical Architectures, Data Processing, and System Efficiency
Abstract
The rapid development of autonomous vehicles (AVs) has transformed the transportation sector, promising significant improvements in safety, efficiency, and convenience. However, the successful deployment of AVs depends on the ability to process vast amounts of data in real-time, ensuring swift decision-making and robust system performance. Edge computing has emerged as a critical technology in addressing these requirements by bringing computational resources closer to the data source, and thus, reducing latency and enhancing data processing capabilities. This paper explores the integration of edge computing into AV systems, focusing on technical architectures, data processing methodologies, and the resultant system efficiency. The study discusses the various architectural frameworks that facilitate the seamless operation of AVs, including the use of distributed computing nodes and localized data centers. The paper also analyzes the data processing techniques essential for handling the massive datasets generated by AV sensors and the algorithms employed to ensure real-time decision-making. Lastly, impact of edge computing on system efficiency are examined, highlighting improvements in latency, bandwidth usage, and overall vehicle performance. The research aims to provide a detailed understanding of how edge computing can enhance the functionality and reliability of autonomous vehicles, paving the way for their widespread adoption.
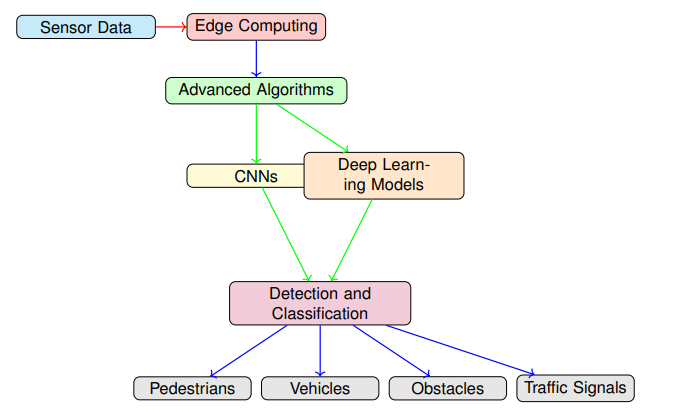
Downloads
Published
How to Cite
Issue
Section
License
Copyright (c) 2023 Applied Research in Artificial Intelligence and Cloud Computing

This work is licensed under a Creative Commons Attribution-NonCommercial-NoDerivatives 4.0 International License.