An Empirical Evaluation Framework for Autonomous Vacuum Cleaners in Industrial and Commercial Settings: A Multi-Metric Approach
Keywords:
Adaptability, Autonomous Vacuum Cleaners, Efficiency, Evaluation Framework, Industrial and Commercial, Performance Metrics, Safety StandardsAbstract
Despite advancements in cleaning automation, there is a noticeable gap in standardized evaluation methods for autonomous vacuum cleaners in industrial and commercial settings. Existing assessments often lack a unified approach, focusing narrowly on either technical capabilities or financial aspects, without integrating both perspectives. This research presents a framework for the evaluation of autonomous vacuum cleaners in industrial and commercial settings, focusing on eight key metrics. These metrics are designed to provide a unified empirical perspective of the vacuum cleaners' performance, operational efficiency, cost, productivity, durability, safety, return on investment, and adaptability. The proposed framework starts with an analysis of cleaning efficiency, examining both the area covered by the cleaners and the quality of cleaning. Advanced image processing techniques are suggested for mapping the area coverage, tailored to different vacuum designs. For assessing cleaning quality, the proposal highlights the potential integration of real-time dirt detection technologies, such as gravimetric sampling and light sensors, to dynamically adapt to varying dirt concentrations and types. Operational efficiency part encompasses the assessment of battery life, charge time, and operational downtime. It advocates for a dual approach of empirical testing and analytical modeling to measure battery life and charge time accurately. The evaluation of operational downtime incorporates tracking of maintenance, charging periods, and other non-operational activities, complemented by predictive modeling for efficient future planning. The financial aspect of the proposed framework encompassed under cost metrics, considers the initial investment, operational and maintenance costs, and potential labor cost savings. This study argues that these cost analysis aids in understanding the long-term financial implications of adopting autonomous vacuum cleaners. Productivity metrics focus on the cleaning speed and the level of autonomy of the vacuum cleaners. Cleaning speed is evaluated using formulas that take into account various environmental factors, while the autonomy level is determined using Sheridan's Levels of Autonomy, which reflects the vacuum's operational independence and its impact on human productivity. Durability, reliability, safety, and compliance are key for vacuum cleaners, evaluated through metrics like Mean Time Between Failures, Mean Time To Repair, Service Life, safety incidents, and adherence to standards and regulations. Lastly, the suggested framework evaluates the vacuum's flexibility and adaptability in different environments, such as various floor types and conditions, highlighting the importance of versatility in autonomous cleaning solutions.
Article history: Received: 01/December /2022; Available online: 07/ February/2023; This work is licensed under a Creative Commons International License.
References
L. D. Knibbs, C. He, C. Duchaine, and L. Morawska, “Vacuum cleaner emissions as a source of indoor exposure to airborne particles and bacteria,” Environ. Sci. Technol., vol. 46, no. 1, pp. 534–542, Jan. 2012.
P. Veerajagadheswar, “TOWARDS DEVELOPING EFFECIENT AREA COVERAGE APPROACH IN DOMESTIC ROBOTS,” 2019.
L. Harmer, T. Cooper, T. Fisher, G. Salvia, and C. Barr, “Design, Dirt and Disposal: Influences on the maintenance of vacuum cleaners,” J. Clean. Prod., vol. 228, pp. 1176–1186, Aug. 2019.
S. Tina Monteiro, “Real Time Measurement of Dirt Pick-up by a Robotic Vacuum Cleaner using Light Sensing Technology,” 2018.
X. Hu, L. Xu, X. Lin, and M. Pecht, “Battery lifetime prognostics,” Joule, vol. 4, no. 2, pp. 310–346, Feb. 2020.
J. L. Sullivan and L. Gaines, “A review of battery life-cycle analysis : state of knowledge and critical needs,” Argonne National Lab. (ANL), Argonne, IL (United States), ANL/ESD/10-7, Dec. 2010.
M. Tomy, B. Lacerda, N. Hawes, and J. L. Wyatt, “Battery Charge Scheduling in Long-Life Autonomous Mobile Robots,” in 2019 European Conference on Mobile Robots (ECMR), 2019, pp. 1–6.
Z. Cheng, X. Fu, J. Wang, and X. Xu, “Research on robot charging strategy based on the scheduling algorithm of minimum encounter time,” J. Oper. Res. Soc., vol. 72, no. 1, pp. 237–245, Jan. 2021.
H. Shakhawat Hossen Prayash, M. R. Shaharear, M. F. Islam, S. Islam, N. Hossain, and S. Datta, “Designing and Optimization of An Autonomous Vacuum Floor Cleaning Robot,” in 2019 IEEE International Conference on Robotics, Automation, Artificial-intelligence and Internet-of-Things (RAAICON), 2019, pp. 25–30.
A. Eren and H. Doğan, “Design and implementation of a cost effective vacuum cleaner robot,” Turk. J. Eng. Environ. Sci., vol. 6, no. 2, pp. 166–177, Apr. 2021.
P. S. Adithya, R. Tejas, V. Sai Varun, and B. N. Prashanth, “Design and Development of Automatic Cleaning and Mopping Robot,” IOP Conf. Ser.: Mater. Sci. Eng., vol. 577, no. 1, p. 012126, Nov. 2019.
M. Vagia, A. A. Transeth, and S. A. Fjerdingen, “A literature review on the levels of automation during the years. What are the different taxonomies that have been proposed?,” Appl. Ergon., vol. 53, pp. 190–202, Mar. 2016.
K. Grythe, T. A. Reinen, and A. A. Transeth, “Autonomy levels versus communication in an underwater environment,” in OCEANS 2015 - Genova, 2015, pp. 1–8.
W. Torell and V. Avelar, “Mean time between failure: Explanation and standards,” 2004. [Online]. Available: https://citeseerx.ist.psu.edu/document?repid=rep1&type=pdf&doi=c81d735b57677b4fc527c7b545b5d0096fcb359f.
Z. Dong, L. Chuan, L. Yongxiang, and G. Zhiqi, “A system’s mean time to repair allocation method based on the time factors,” Qual. Reliab. Eng. Int., vol. 30, no. 2, pp. 247–256, Mar. 2014.
T. E. Wing and L. H. Crow, “A model for mean-time-to-repair and mean-logistics-delay-time at the system level,” in Annual Proceedings on Reliability and Maintainability Symposium, 1990, pp. 389–393.
K. Hattori, S. Morford, and D. T. Delaney, “How to obtain maximum underwriters laboratories coverage,” J. Vinyl Addit. Technol., vol. 1, no. 3, pp. 170–173, Sep. 1995.
S. Interconnection, “Underwriters Laboratories,” nrel.gov.
A. De Santis, B. Siciliano, A. De Luca, and A. Bicchi, “An atlas of physical human–robot interaction,” Mechanism and Machine Theory, vol. 43, no. 3, pp. 253–270, Mar. 2008.
M. Kukde, S. Nagpurkar, and A. Dhakulkar, “Automatic & manual vacuum cleaning robot,” Research, 2018.
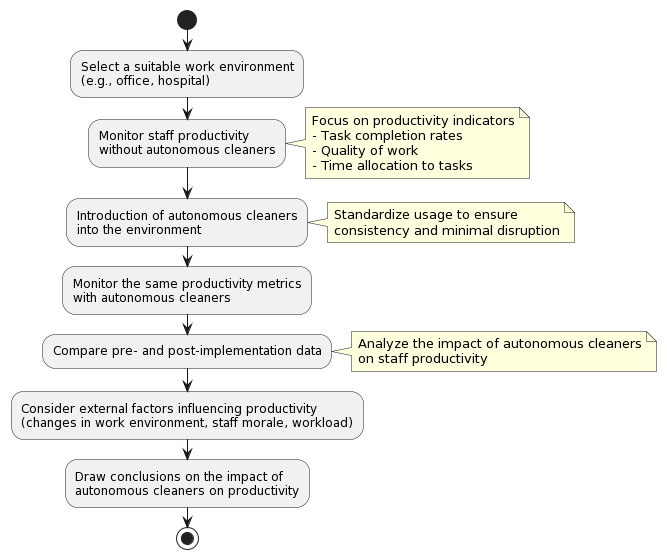
Downloads
Published
How to Cite
Issue
Section
License
Copyright (c) 2023 ResearchBerg

This work is licensed under a Creative Commons Attribution-NonCommercial-NoDerivatives 4.0 International License.
Creative Commons licenses are used to publish Open Access articles, which provide the legal basis for users to access, distribute, and reuse the content. EQME allows authors to apply one of the following Creative Commons licenses to their work, each of which affords readers distinct rights in terms of commercial use and the capacity to create derivative versions:
CC-BY (Creative Commons Attribution License)
CC-BY-NC-ND (Creative Commons Non-Commercial No Derivatives License)
CC-BY-NC-SA (Creative Commons Non-Commercial Share-a-like)
In each situation, the creator must be given credit, and if derivative versions of the work are created, the alterations must be noted.