Leveraging Predictive Modeling, Machine Learning Personalization, NLP Customer Support, and AI Chatbots to Increase Customer Loyalty
Keywords:
AI, Business, Customer loyalty, Machine learning, NLP, Predictive modelingAbstract
AI, ML, and NLP are profoundly altering the way organizations work. With the increasing influx of data and the development of AI systems to understand it in order to solve business challenges, the excitement surrounding AI has grown. Massive datasets, computer capacity, improved algorithms, accessible algorithm libraries, and frameworks have compelled today's organizations to use AI to enhance their operations and profits. These technologies aid every kind of industry, from agriculture to finance. More specifically, AI and ML, and NLP are assisting organizations in areas such as customer service, predictive modeling, customer personalization, picture identification, sentiment analysis, offline and online document processing. The purpose of this study was twofold. We first review the several applications of AI in business and then empirically test whether these applications increase customer loyalty using the datasets of 910 firms around the world. The datasets include the integration scores of four different AI features, namely, AI-powered customer service, predictive modeling, ML-powered personalization, and natural language processing integration. The target is the customer loyalty measure as binary. All the features are measured on a 5-pint Likert scale. We applied six different supervised machine learning algorithms, namely, Logistic regression, KNN, SVM, Decision Tree, Random Forest, and Ada boost Classifiers. the performance of each algorithm was evaluated using confusion matrices and ROC curves. The Ada boost and logistic classifiers performed better with test accuracies of 0.639 and 0.631, respectively. The decision tree and KNN had the performance with accuracies of 0.532 and 0.570, respectively. The findings of this study highlight that by incorporating AI, ML, and NLP, businesses may analyze data to uncover what's useful, gaining valuable insights that can be used to automate processes and drive business strategies. As a result, firms that wish to remain competitive and increase customer loyalty should adopt them.
References
Abbet, C., M’hamdi, M., Giannakopoulos, A., West, R., Hossmann, A., Baeriswyl, M., & Musat, C. (2018). Churn Intent Detection in Multilingual Chatbot Conversations and Social Media. In arXiv [cs.CL]. arXiv. http://arxiv.org/abs/1808.08432
Berry, C., Wang, H., & Hu, S. J. (2013). Product architecting for personalization. Journal of Manufacturing Systems, 32(3), 404–411.
Bleier, A., De Keyser, A., & Verleye, K. (2018). Customer Engagement Through Personalization and Customization. In R. W. Palmatier, V. Kumar, & C. M. Harmeling (Eds.), Customer Engagement Marketing (pp. 75–94). Springer International Publishing.
Bradlow, E. T., Gangwar, M., Kopalle, P., & Voleti, S. (2017). The Role of Big Data and Predictive Analytics in Retailing. Journal of Retailing, 93(1), 79–95.
Burez, J., & Van den Poel, D. (2009). Handling class imbalance in customer churn prediction. Expert Systems with Applications, 36(3, Part 1), 4626–4636.
Cheng, Y., & Jiang, H. (2020). How Do AI-driven Chatbots Impact User Experience? Examining Gratifications, Perceived Privacy Risk, Satisfaction, Loyalty, and Continued Use. Journal of Broadcasting & Electronic Media, 64(4), 592–614.
Drus, Z., & Khalid, H. (2019). Sentiment Analysis in Social Media and Its Application: Systematic Literature Review. Procedia Computer Science, 161, 707–714.
Duan, Edwards, & Dwivedi. (2019). Artificial intelligence for decision making in the era of Big Data–evolution, challenges and research agenda. International Journal of Information Technology and Management. https://www.sciencedirect.com/science/article/pii/S0268401219300581
Eckerson. (2007). Predictive analytics. Extending the Value of Your Data. http://download.101com.com/pub/tdwi/files/pa_report_q107_f.pdf
Farzindar, A., & Inkpen, D. (2015). Natural Language Processing for Social Media. Synthesis Lectures on Human Language Technologies, 8(2), 1–166.
Følstad, A., & Skjuve, M. (2019). Chatbots for customer service: user experience and motivation. Proceedings of the 1st International Conference on Conversational User Interfaces, 1–9.
Hadden, J., Tiwari, A., Roy, R., & Ruta, D. (2007). Computer assisted customer churn management: State-of-the-art and future trends. Computers & Operations Research, 34(10), 2902–2917.
Hasan, M. R., Maliha, M., & Arifuzzaman, M. (2019). Sentiment Analysis with NLP on Twitter Data. 2019 International Conference on Computer, Communication, Chemical, Materials and Electronic Engineering (IC4ME2), 1–4.
Kuligowska, & Lasek. (2011). Virtual assistants support customer relations and business processes. The 10th International Conference On. http://wne.kuligowska.com/papers/Kuligowska_Lasek_Virtual_assistants_2011.pdf
Kulkarni, A., & Shivananda, A. (2019). Natural Language Processing Recipes. Apress.
Lu, J., Zhang, Q., & Zhang, G. (2012). Recommender Systems. World Scientific. https://www.worldscientific.com/worldscibooks/10.1142/11947
Nanli, Z., Ping, Z., Weiguo, L., & Meng, C. (2012). Sentiment analysis: A literature review. 2012 International Symposium on Management of Technology (ISMOT), 572–576.
Nordheim, C. B., Følstad, A., & Bjørkli, C. A. (2019). An Initial Model of Trust in Chatbots for Customer Service—Findings from a Questionnaire Study. Interacting with Computers, 31(3), 317–335.
Piccoli, G., Lui, T.-W., & Grün, B. (2017). The impact of IT-enabled customer service systems on service personalization, customer service perceptions, and hotel performance. Tourism Management, 59, 349–362.
Prajwal, S. V., Mamatha, G., Ravi, P., Manoj, D., & Joisa, S. K. (2019). Universal Semantic Web Assistant based on Sequence to Sequence Model and Natural Language Understanding. 2019 9th International Conference on Advances in Computing and Communication (ICACC), 110–115.
Ramaswamy, S., & DeClerck, N. (2018). Customer Perception Analysis Using Deep Learning and NLP. Procedia Computer Science, 140, 170–178.
Rathee, V. S., Sidky, H., & Sikora, B. J. (2018). Role of associative charging in the entropy–energy balance of polyelectrolyte complexes. Journal of the American. https://pubs.acs.org/doi/abs/10.1021/jacs.8b08649
Rathee, Vikramjit S., Sidky, H., Sikora, B. J., & Whitmer, J. K. (2019). Explicit Ion Effects on the Charge and Conformation of Weak Polyelectrolytes. Polymers, 11(1). https://doi.org/10.3390/polym11010183
Rathee, Vikramjit S., Zervoudakis, A. J., Sidky, H., Sikora, B. J., & Whitmer, J. K. (2018). Weak polyelectrolyte complexation driven by associative charging. The Journal of Chemical Physics, 148(11), 114901.
Rese, Ganster, & Baier. (2020). Chatbots in retailers’ customer communication: How to measure their acceptance? Journal of Retailing and Consumer Services. https://www.sciencedirect.com/science/article/pii/S0969698920308286
Rokade, & Aruna. (2019). Business intelligence analytics using sentiment analysis-a survey. International Journal of Electrical and Computer Engineering (IJECE). https://www.academia.edu/download/65965681/11092.pdf
Rossmann, A., Zimmermann, A., & Hertweck, D. (2020). The Impact of Chatbots on Customer Service Performance. Advances in the Human Side of Service Engineering, 237–243.
Sagiroglu, S., & Sinanc, D. (2013). Big data: A review. 2013 International Conference on Collaboration Technologies and Systems (CTS), 42–47.
Salinca, A. (2015). Business Reviews Classification Using Sentiment Analysis. 2015 17th International Symposium on Symbolic and Numeric Algorithms for Scientific Computing (SYNASC), 247–250.
Sanguansat, P. (2016). Paragraph2Vec-based sentiment analysis on social media for business in Thailand. 2016 8th International Conference on Knowledge and Smart Technology (KST), 175–178.
Seljan, & Dunđer. (2014). Combined automatic speech recognition and machine translation in business correspondence domain for english-croatian. International Journal of Industrial and Systems Engineering. https://citeseerx.ist.psu.edu/viewdoc/download?doi=10.1.1.667.819&rep=rep1&type=pdf
Shafin, M. A., Hasan, M. M., Alam, M. R., Mithu, M. A., Nur, A. U., & Faruk, M. O. (2020). Product Review Sentiment Analysis by Using NLP and Machine Learning in Bangla Language. 2020 23rd International Conference on Computer and Information Technology (ICCIT), 1–5.
Shani, G., & Gunawardana, A. (2011). Evaluating Recommendation Systems. In F. Ricci, L. Rokach, B. Shapira, & P. B. Kantor (Eds.), Recommender Systems Handbook (pp. 257–297). Springer US.
Sheehan, B., Jin, H. S., & Gottlieb, U. (2020). Customer service chatbots: Anthropomorphism and adoption. Journal of Business Research, 115, 14–24.
Sidky, H., Colón, Y. J., Helfferich, J., Sikora, B. J., Bezik, C., Chu, W., Giberti, F., Guo, A. Z., Jiang, X., Lequieu, J., Li, J., Moller, J., Quevillon, M. J., Rahimi, M., Ramezani-Dakhel, H., Rathee, V. S., Reid, D. R., Sevgen, E., Thapar, V., … de Pablo, J. J. (2018). SSAGES: Software Suite for Advanced General Ensemble Simulations. The Journal of Chemical Physics, 148(4), 044104.
Strickland, J. (2014). Predictive Modeling and Analytics. Lulu.com.
Trivedi, J. (2019). Examining the Customer Experience of Using Banking Chatbots and Its Impact on Brand Love: The Moderating Role of Perceived Risk. Journal of Internet Commerce, 18(1), 91–111.
Trivedi, S., & Patel, N. (2020a). Clustering Students Based on Virtual Learning Engagement, Digital Skills, and E-learning Infrastructure: Applications of K-means, DBSCAN, Hierarchical, and Affinity Propagation Clustering. Sage Science Review of Educational Technology, 3(1), 1–13.
Trivedi, S., & Patel, N. (2020b). The Impact of Artificial Intelligence Integration on Minimizing Patient Wait Time in Hospitals. Researchberg Review of Science and Technology, 3(1), 21–35.
Tsai, C.-H. (2016). A Fuzzy-Based Personalized Recommender System for Local Businesses. Proceedings of the 27th ACM Conference on Hypertext and Social Media, 297–302.
van Eeuwen, M. (2017). Mobile conversational commerce: messenger chatbots as the next interface between businesses and consumers [University of Twente]. http://essay.utwente.nl/71706/
Verhagen, Van Nes, & Feldberg. (2014). Virtual customer service agents: Using social presence and personalization to shape online service encounters. Journal of Computational Systems Biology. https://academic.oup.com/jcmc/article-abstract/19/3/529/4067591
Vesanen, J., & Raulas, M. (2006). Building bridges for personalization: A process model for marketing. Journal of Interactive Marketing, 20(1), 5–20.
Vossen, G. (2014). Big data as the new enabler in business and other intelligence. Vietnam Journal of Computer Science, 1(1), 3–14.
Walter, F. E., Battiston, S., & Schweitzer, F. (2008). A model of a trust-based recommendation system on a social network. Autonomous Agents and Multi-Agent Systems, 16(1), 57–74.
Wassouf, W. N., Alkhatib, R., Salloum, K., & Balloul, S. (2020). Predictive analytics using big data for increased customer loyalty: Syriatel Telecom Company case study. Journal of Big Data, 7(1), 29.
Wu, J., Wang, L., & Liu, Y. (2018). Research on financial advertisement personalised recommendation method based on customer segmentation. International Journal of Wireless and Mobile Computing, 14(1), 97.
Zaki, Kandeil, & Neely. (2016). The fallacy of the net promoter score: Customer loyalty predictive model. Cambridge Anthropology: A Journal of the Department of Social Anthropology, Cambridge University. https://cambridgeservicealliance.eng.cam.ac.uk/system/files/documents/2016OctoberPaper_FallacyoftheNetPromoterScore.pdf
Zoghbi, Heyman, & Gomez. (2016). Fashion meets computer vision and nlp at e-commerce search. International Journal of High Risk Behaviors & Addiction. https://lirias.kuleuven.be/1656599?limo=0
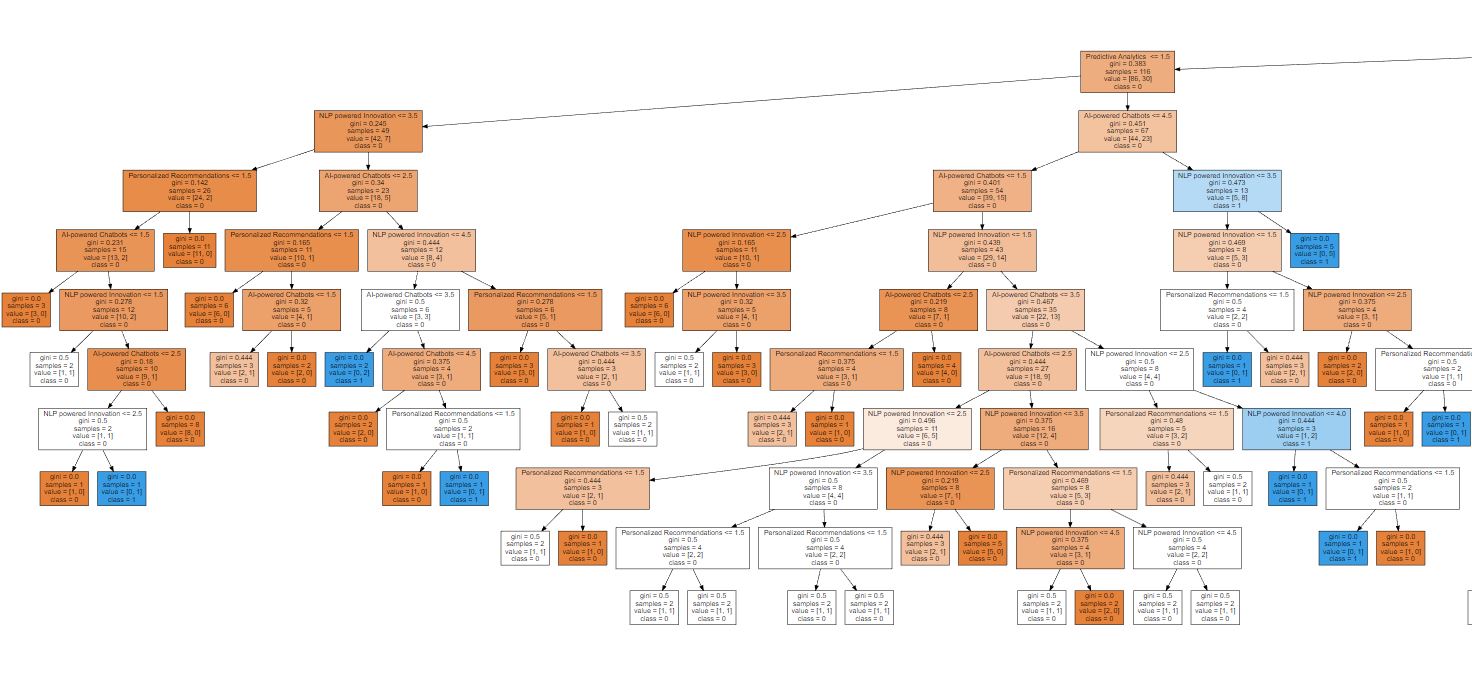
Downloads
Published
How to Cite
Issue
Section
License
Copyright (c) 2020 Author

This work is licensed under a Creative Commons Attribution-NonCommercial-NoDerivatives 4.0 International License.
Creative Commons licenses are used to publish Open Access articles, which provide the legal basis for users to access, distribute, and reuse the content. EQME allows authors to apply one of the following Creative Commons licenses to their work, each of which affords readers distinct rights in terms of commercial use and the capacity to create derivative versions:
CC-BY (Creative Commons Attribution License)
CC-BY-NC-ND (Creative Commons Non-Commercial No Derivatives License)
CC-BY-NC-SA (Creative Commons Non-Commercial Share-a-like)
In each situation, the creator must be given credit, and if derivative versions of the work are created, the alterations must be noted.