Personalized Employee Training Based on Learning Styles Using Unsupervised Machine Learning
Keywords:
Employee, FSLSM, Learning styles, Machine learning, Personalized trainingAbstract
Advancement in technology, artificial intelligence, and machine learning have resulted in an explosion in the creation of tech-enabled training solutions over the past decade, contributing to the popularity of personalized learning. This research advocates for a transition away from archaic, rote learning paradigms and toward individualized employee learning experiences in which instructional styles and training tactics are tailored to the requirements of each individual employee rather than standard lesson preparation that exist today. This technique can also foster enjoyable and engaging training environments that benefit both employees and the organizations. We applied unsupervised machine learning algorithm, namely, K-means, and Hierarchical clustering algorithms to classify 1000 employees into different clusters based on the Felder-Silverman Learning Styles Model (FSLSM). As expected, no one of the employees could be precisely classified into a single category, and they demonstrated a variety of learning methods and tactics. The experiments showed 3 significant clusters across the different pairs of Processing, Input, Understanding, and Perception dimensions of the FSLSM. The findings suggest that employees can be grouped into at least 3 clusters to create personalized training materials and approaches for each group. We also discussed suitable instruction techniques, contents, and paths for each cluster. The proposed model and the findings would work in both digital and offline settings.
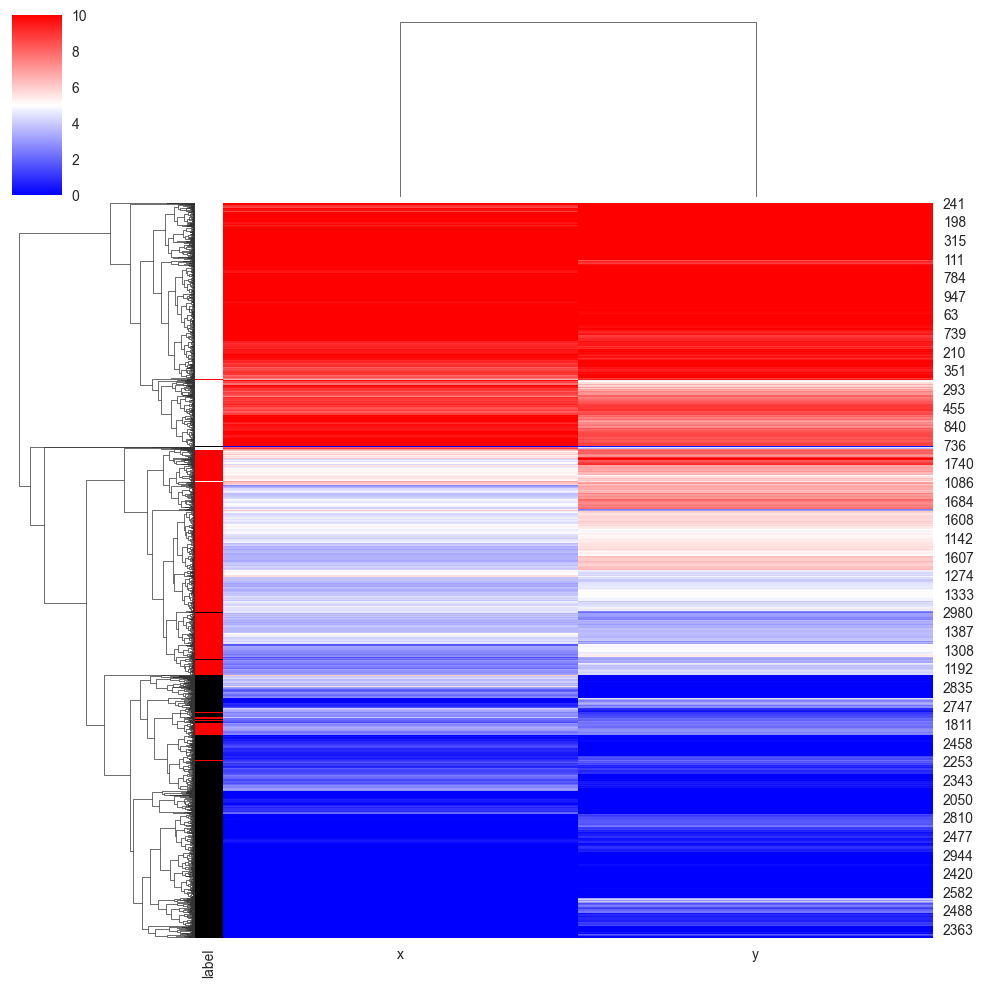
Downloads
Published
How to Cite
Issue
Section
License
Publication Licensing Terms:
Copyright: Authors retain copyright, granting the journal first publication under a Creative Commons Attribution (CC BY) license.
Licensing: The CC BY license allows any use with proper attribution and a link to the journal's website.
Author's Warranties: Authors confirm their work is original and does not violate any third-party rights.
Reuse and Distribution: Authors are free to share their work online after publication.
Attribution: Users must credit the work as specified under the CC BY license without suggesting author endorsement.
Responsibility: Authors bear responsibility for copyright infringement issues.